Machine learning in programmatic advertising has existed from the very dawn of algorithmic buying. It always played a huge role in amplifying the effectiveness of the ad campaigns, which happened thanks to the optimization of various media-buying processes, including the aspects of creative placements, bidding dynamics, and even determining the optimal ad frequency.
For almost a decade, the role of ML media buying has been pretty straightforward; however, as we marched into a completely new era in which technologies like ChatGPT revolutionized AdTech, the expectations of advertisers grew along with the expanding capacities of ad platforms.
So, what can smart algorithms do for media-buying enhancement in 2024, and how can we benefit from opening opportunities?
The role of machine learning in RTB
Machine Learning is a subset of AI that focuses on developing algorithms and statistical models that enable computers to learn from data without involving programming. It is a practical application of AI, using data-driven models for accurate prediction and optimization of advertising campaign performance.
In contrast, artificial intelligence refers to the broader concept of computer systems that can mimic human intelligence, including tasks such as speech recognition, problem-solving, and decision-making.
ML programmatic advertising algorithms can work in numerous ways. For instance, thanks to these algorithms, advertising platforms significantly improve the outcomes of real-time bidding.
- Predicting the likelihood of winning. ML predictors are integrated into platforms to enhance the inventory purchasing process. These predictors are activated during the ad impression acquisition phase. Using machine learning algorithms, they predict the likelihood of a desired action (such as a click or purchase) based on the advertiser's specific goals and objectives.
- Making data-driven decisions. Leveraging a pre-built model derived from user data provided by the ad platform or RTB platform during the impression acquisition stage, the algorithm decides whether to bid for the impression. If machine learning algorithms for programmatic advertising determine that the likelihood of a desired action is high, they decide to bid for the ad. In a similar way, demand-side platforms that operate on a CPM advertising model (cost per 1000 impressions) evaluate whether to participate in the auction for the ad impression, whether to bid higher or lower to win the auction, and many other strategic decisions. This ensures that every bid is like a carefully calculated gamble, maximizing the chances of success.
- Determining bid amount. When the machine learning tools for media buying decide to participate in the bidding for a particular impression (based on the advertiser’s campaign configurations), it also determines what kind of bid to offer in the RTB auction in order to win it. On the contrary, if the bid is too big, the DSP will win in the auction, albeit at a cost that is way too high. In order to prevent overspending, DSPs deploy bid optimization algorithms based on ML.
Key benefits of machine learning in programmatic advertising
So far, we’ve discussed how machine learning is integrated into the RTB process in order to enhance it. Still, the bidding process is not the only thing that can be enhanced thanks to the smart algorithm implementation. Ad platforms can benefit from it in a myriad of ways, especially when it comes to improving targeting precision, analytics, or even fraud detection.
Read here about programmatic vs RTB.
Machine learning programmatic advertising has the potential to:
Personalize user experience through better targeting
Today, advertisers can tailor ad copy to the specific language nuances of a region by using relevant key phrases and idioms that make the ad clearer, more relatable, and more engaging for the target audience. Additionally, the user's location can be leveraged by AI tools to target ads at moments when they are near a physical store, increasing the chances of making a purchase. This will be specifically relevant for the geo-targeting campaigns.
Moreover, as popular alternatives to cookie-based targeting, experts recommend contextual targeting, which can be significantly enhanced by implementing AI and ML algorithms to more accurately determine the context of the page where the ad will be placed.
Reduce the budget for the advertiser
Again, this happens thanks to the optimization of the bid amount that we mentioned before. The ML model enables a reduction in conversion costs by automatically adjusting (increasing or decreasing) the bid based on the likelihood of achieving a conversion (or winning in the auction). This way, advertisers can be sure that they win the impressions, yet they don’t need to worry about spending too much.
Improve analytics
On certain platforms, advertisers can even gain in-depth insights into user behavior in order to generate actionable insights that help evaluate campaign effectiveness. This data-driven approach, coupled with programmatic advertising and machine learning, allows advertisers to make informed decisions and optimize their campaigns for better outcomes.
Improve creatives
A lot of ad platforms implement machine learning for advertising when it comes to creatives. Namely, ML can improve the rendering of different ad creative sizes and automatically optimize them to achieve maximum effectiveness.
Detect and prevent ad fraud
Normally, ad platforms implement two-layered protection against ad fraud. The first includes in-house ML-based ad fraud detection and prevention. The second layer is a collaboration with anti-fraud detection companies (e.g., Pixalate), which constantly develop their own ML models for the deepest threat search and prevention.
Real-world examples of implementing ML in programmatic
Machine learning in programmatic ad buying can elicit spectacular results, and we are going to review some of them in detail.
Case 1 – Reducing CPM
Some platforms like SmartyAds employ machine learning tools for media buying like Adaptive CPM, which keeps the bid amount at the optimal level for advertisers.
The challenge
To win impressions at the RTB auction at an optimal level (without overpaying).
The solution
ML was implemented in the bidding process.
The result
The adaptive CPM feature from SmartyAds was implemented into the DSP to analyze bidding patterns efficiently and develop the most effective strategy for advertisers to win impressions while keeping the CPM level high enough to win in the auction yet low enough to prevent paying too much. As a result, the win rate for studied campaigns increased from 20% to 40%.
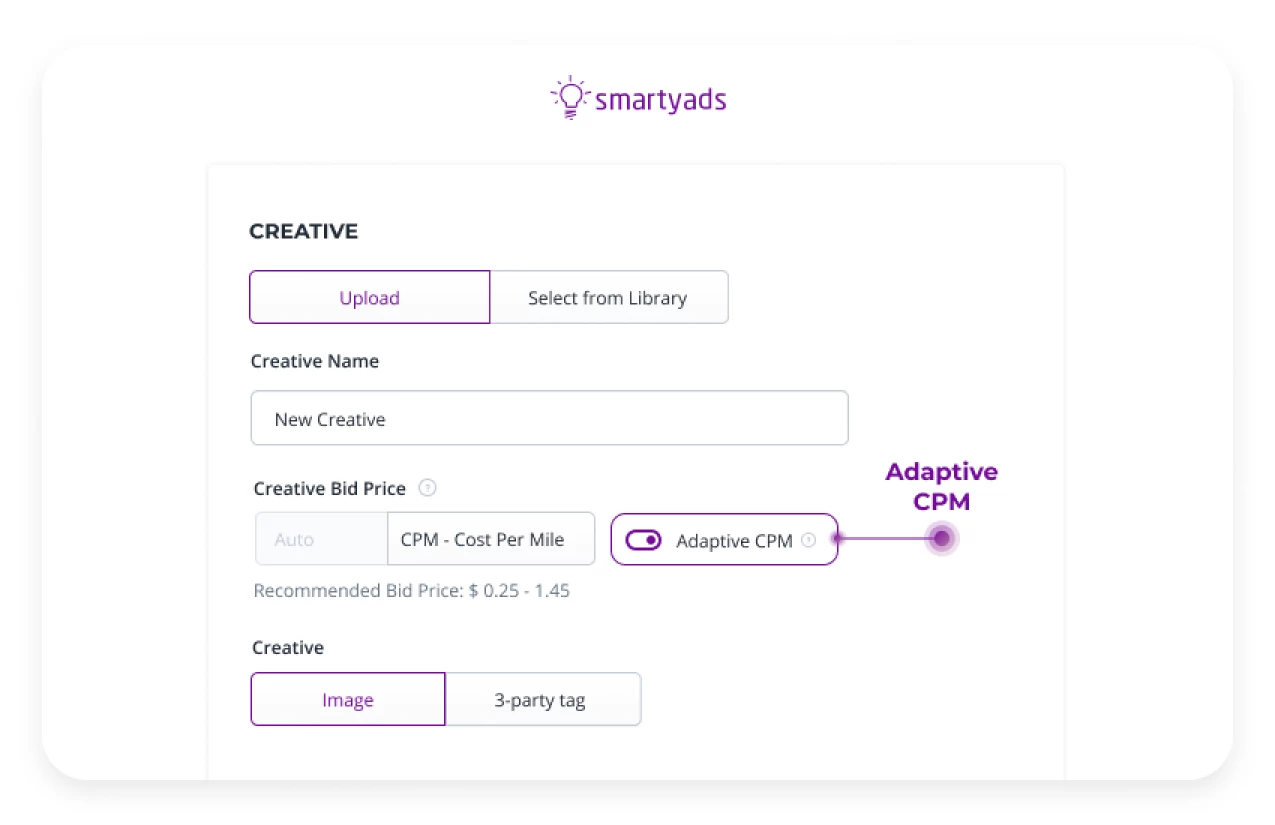
Case 2 – Improved targeting
Political campaigns can be especially dependent on precise targeting, which is why Local Majority selected SmartyAds DSP to reach the right audience.
The challenge
Local Majority strived to reach increased visibility in particular areas.
The solution
The client was encouraged to apply smart ML-driven targeting mechanisms available at SmartyAds DSP.
The result
The campaign outperformed the client's expectations, generating significant impressions and clicks in the targeted geographical area. This resulted in a CTR boost of up to 27% and much more.
Case 3 – Higher ad click-through rates
Every advertiser can achieve better results by setting up their own rules on demand-side platforms because each advertiser has individual requirements regarding traffic.
The challenge
To let advertisers prioritize the sources that have the highest CTRs and to reduce impressions on those that have low CTRs.
The solution
SmartyAds DSP was equipped with ML programmatic advertising optimization rules to let advertisers optimize their traffic individually.
The result
Advertisers who implemented this feature could lift CTRs from 10% to 30%. Also, they could effectively reduce budget waste and achieve better campaign performance.
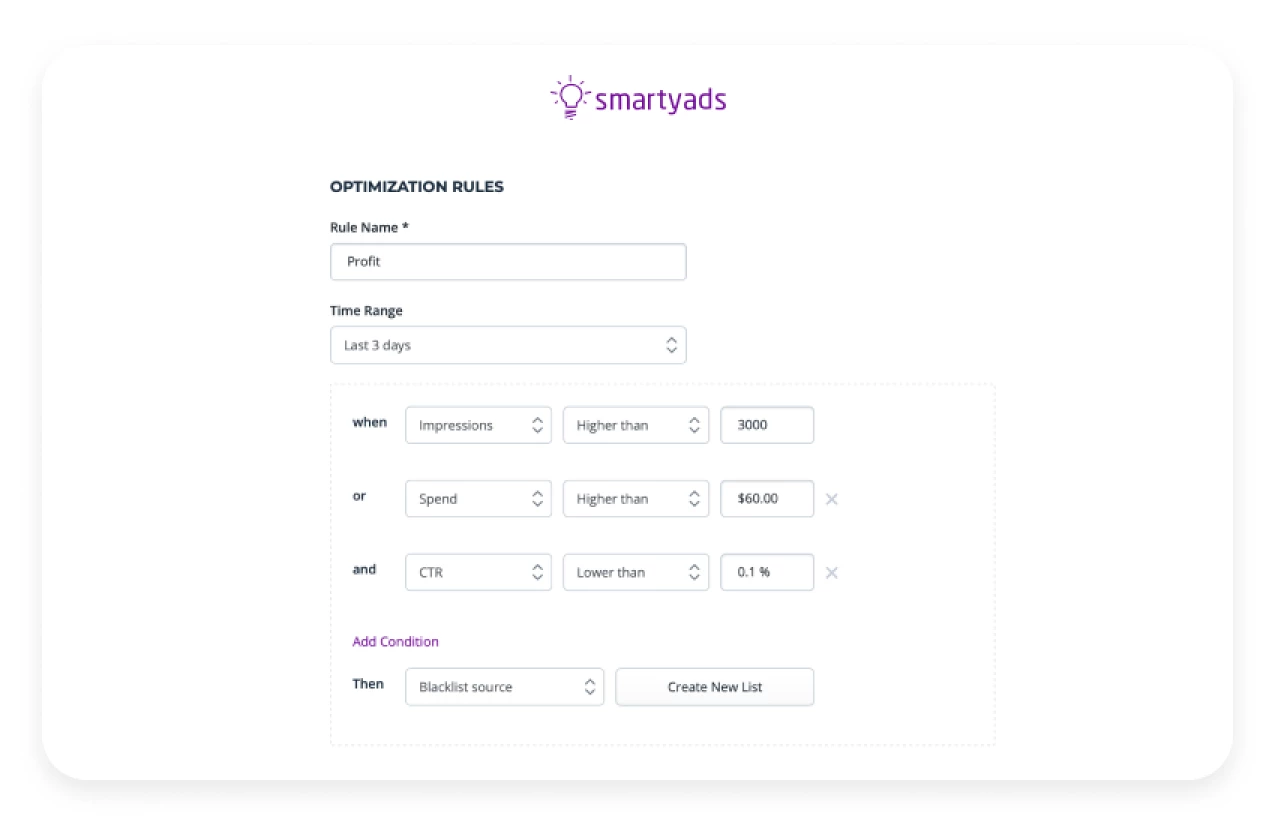
Programmatic advertising and machine learning: challenges and solutions
This table outlines various challenges and how machine learning can provide solutions to enhance efficiency, effectiveness, and precision in programmatic advertising. Note that when it comes to the implementation of machine learning for ad serving, capacities range from platform to platform and will depend on what particular vendor has on offer.
Challenge | ML solution |
Audience targeting | ML can analyze large datasets to identify and target specific audience segments with high precision. |
Ad fraud detection
| ML algorithms can detect patterns indicative of fraudulent activities and prevent ad fraud in real time. |
Bid optimization
| ML can optimize bidding strategies by predicting the best bid prices based on historical data and trends. |
Personalization
| ML can deliver personalized ads by analyzing user behavior and preferences to increase engagement.
|
Creative optimization
| ML can test and optimize different ad creatives to determine which one performs best with specific audiences.
|
Predictive analytics
| ML can predict future trends and user behavior to inform ad strategies and improve campaign outcomes.
|
Customer journey mapping
| ML can analyze user interactions across various touchpoints to create comprehensive customer journey maps.
|
Real-time ad placement
| ML can decide the best time and place to display ads for maximum impact in real time.
|
Budget allocation
| ML can allocate budgets efficiently across channels and campaigns to maximize ROI.
|
Performance tracking and reporting
| ML and programmatic advertising can automate the tracking and reporting of ad performance metrics, providing insights for optimization.
|
Cross-device tracking
| ML can track user interactions across multiple devices to provide a unified view of user behavior.
|
Contextual advertising
| ML can analyze the context of content to ensure ads are placed in relevant environments.
|
Boost conversions with SmartyAds AI-driven programmatic advertising solutions
Machine learning and programmatic advertising go hand in hand when it comes to SmartyAds DSP, as the above-mentioned case studies prove. In 10+ years, we’ve built an entire infrastructure of programmatic solutions designed to ease the advertising process and boost its efficiency.
Continuous learning and adaptation are essential for growth in the face of constantly changing trends and technologies. It is crucial to find effective solutions to problems that media buyers encounter. Understanding this, we carefully monitor our clients' feedback and search for the most viable technologies based on smart algorithms. These technologies effectively automate media buying and result in enhanced media-buying outcomes.
More illustrative cases of smart algorithm implementation in our DSP (with measurable results) can be found in case studies. Join our DSP to be among the winners who create and promote their campaign with ease and impact!
To wrap it up
Advertisers and marketers have long faced recurring challenges: understanding consumer preferences, expanding ad reach, and enhancing ad efficiency while minimizing costs and manual efforts. ML algorithms for programmatic advertising solve these challenges. Modern DSPs empower advertisers to set customized rules for ad placements and conditions. Leveraging these advanced tools, media buyers can easily achieve optimal results with minimal effort and budget.
Want to leverage ML in programmatic advertising to achieve all your KPIs? Join SmartyAds DSP.