It's fascinating to note that in 1937, Franklin D. Roosevelt's administration managed an unprecedented 29 million record entries, making it the world's first major data project. Fast forward to 2016, global internet traffic exceeded 1 billion terabytes, with 90% of the world's data being generated in just a few years. Looking ahead, the global data storage market is expected to reach $101 billion by 2029. Surprisingly, only 0.5% of the world's data is currently analyzed, and just 5% is structured, highlighting the vast untapped potential hidden within raw data.
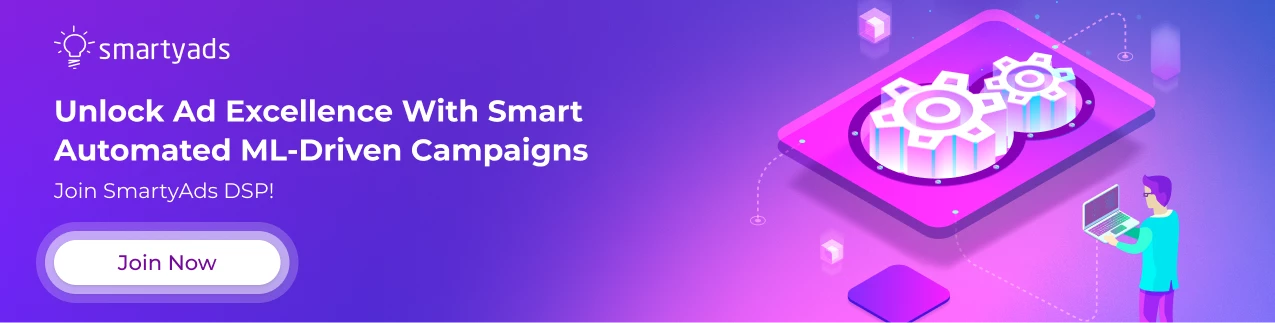
How big data analytics works
Big data analytics definition emphasizes the process of scrutinizing vast datasets to discover patterns and insights. Data mining is super important for spotting trends that help with forecasting. Companies really need to get their data working together to analyze things properly. With some fancy tools like machine learning - think of natural language processing and predictive analytics - we can sift through any kind of data, whether it's organized or a total jumble.
With text analytics, we can extract key information, distill texts to their essence, determine the conversation's vibe, and even obtain answers to specific questions. When it comes to understanding social media's hustle and bustle, we're using cool tactics to see how groups form, who influences who, and how everyone is connected.
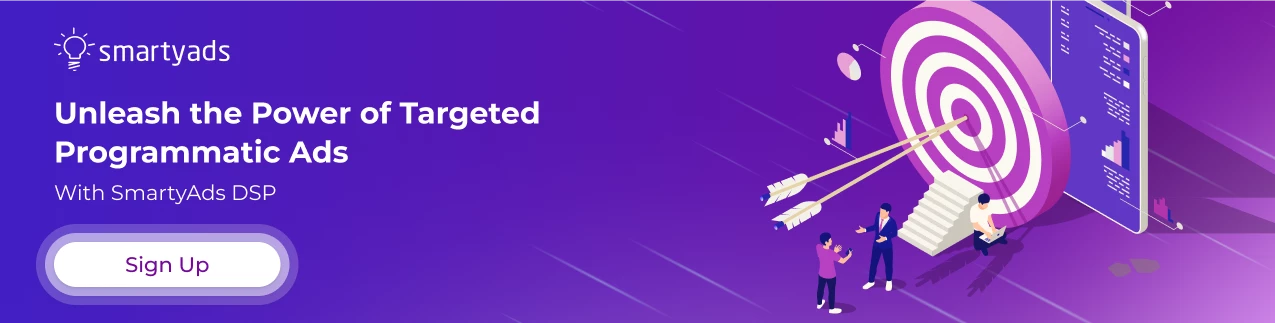
Benefits of big data analytics use
Enhancing decision processes
Big data analytics is changing the game when it comes to making decisions. By digging into huge piles of data, both neat and messy, it helps to uncover really valuable insights. This leads to making much better decisions in the end.
Boosting operational effectiveness
By looking into how things are running, companies can tweak their processes and use their resources smarter. Predicting and preventing problems before they happen helps keep everything running smoothly and efficiently.
Tailoring customer interactions
Using big data, companies can get to know their customers better, crafting personalized experiences that boost happiness and loyalty.
Gaining a market edge
Companies that really tap into the potential of big data analytics gain a competitive edge. They're keen at spotting trends, figuring out what customers really want, and tweaking their operations to deliver top-notch products and services.
Enhancing cost efficiency
By tapping into real-time analytics, companies can find savings by streamlining their supply chains, predicting maintenance needs, and getting demand forecasts right, all of which can boost profits and sustainability.
Elevating risk management
By analyzing trends from past and present data, big data analytics allows companies to spot and lower risks, making their operations more secure.
Main data analysis methods
Descriptive analytics
Descriptive analytics is all about digging into past data to spot trends and understand what's been happening. It helps us by breaking down key performance indicators and metrics into easy-to-understand reports and dashboards. Think of it as a way to get a clear picture of business operations, such as figuring out which products are selling best or spotting seasonal trends in sales data.
Diagnostic analytics
Diagnostic analytics digs into why things happened, looking at data to spot causes and patterns. It's key for businesses to understand deviations and uncover underlying links.
Predictive analytics
Predictive analytics leverages data and models to predict the future by spotting trends and patterns. It's handy for forecasting customer behavior, market trends, and identifying risks. Imagine a retailer using this method to figure out how much of a product they'll need in the future, based on their past sales and other factors like the weather or the state of the economy.
Prescriptive analytics
Prescriptive analytics uses algorithms to suggest the best steps for the ideal results. It's great for tricky decisions, like improving supply chains or tailoring online shopping deals.
Machine learning
Machine learning is a key piece of AI that helps sift through heaps of data to spot patterns, categorize information, and make guesses about what might happen next. It's behind things like those spot-on show recommendations, uncovering dodgy transactions, and figuring out the mood of social media chatter.
Text analytics
Text analytics, or text mining, is all about sifting through stuff like social media posts, customer feedback, and emails to figure out what people are really saying. It’s like detective work, using cool tools like natural language processing to spot trends, feelings, and opinions hidden in all those words. This way, businesses can get the lowdown on what's buzzing and use that to make smart moves.
Cluster analysis
Cluster analysis is a technique that bunches together similar pieces of data, making it easier to spot natural clusters within a dataset. This method is especially handy when it comes to dividing up customers into different segments or for digging into market research.
What Is big data analytics process?
Big data analytics involves breaking down data into separate elements for detailed examination. The process includes obtaining raw data and delivering the desired output. The lifecycle of data analytics depends on the business case and can be broken down into major phases.
Data specification
Specify the needed data and its sources, set up a file naming and storage system, establish clear data collection requirements, decide on the database or sources to use, and ensure collaborative work to avoid data duplication and missing gaps.
Data acquisition
During the second stage of digital marketing, data about the user is collected, including locations, status updates, image downloads, links, interactions, search history, comments, etc. The system also collects data on the user's page engagement, clicks, topics, interests, and other information relevant for data analysis.
Data processing
After collecting data, it is organized into categories using digital statistical software or spreadsheets.
Data validation and cleansing
In data analytics, this step involves cleaning and filtering to ensure high data quality. The system identifies and removes incomplete data, errors, duplications, and inaccuracies.
Data analysis
After refining and distilling, the system will employ exploratory analysis of the data to understand its characteristics, define the causes of the event, assess assumptions, and pinpoint key features for further analysis.
Data modeling
Key features generated in the previous stage are used to feed the mathematical formulas and machine learning algorithms to formulate a model. Algorithms will help to identify the cause-and-effect relationships, mutual relationships and connections among variables.
Generation of the output
The system will generate a model that will imitate a real business problem and set a task, for example, to predict the outcome of the action. What follows next is training and testing possible solutions to predict the behavior of the variables.
Data visualization and presentation
Once the data is analyzed and the output is generated, it may be presented in various forms, such as tables, charts, visual plots or graphs. The output will present the results of data analysis as well as valuable insights that were not possible to obtain without machine processing.
The Seven "V"s of Big Data?
Big data and data analytics create both opportunities and challenges. For proper big data analysis, it is important to know the characteristics of big data that have been traditionally defined by seven Vs: volume, velocity, variety, veracity, value, variability and visualization.
- Volume: the actual amount of data or how much data weights. An average PC computer in 2000 had 10GB of storage, whereas today, typical PC users enjoy 500GB to 1TB of hard drive memory. For comparison, Google processes somewhere around 3.5 billion search requests per day and claims to store 10 to 15 exabytes of data (1 exabyte equals 1 billion terabytes).
- Velocity: the rate at which data is generated and the speed at which it must be processed. Nowadays, with torrents of data coming from sensors and devices, data has to be analyzed immediately. Machines produce multiple outputs in milliseconds, and the highest data velocity is considered nearly real-time evaluation and action.
- Variety: data types. Data is classified into structured, semi-structured, and unstructured. Structured data is presented by numerical information in the tabular formats. Text, email, financial transactions, digital images, and video files are examples of unstructured data that require structural organization.
- Veracity: trustworthiness and consistency of the data. To produce credible results, data must possess quality. If the data is incomplete, ambiguous or contain errors or duplicates, it cannot be analyzed or processed correctly.
- Value: whether data can be easily accessed to deliver quality analytics. If the data is valuable, it delivers actionable insights, enables informed decisions, and provides significant details.
- Variability: constant change of data’s meaning. What a piece of data means today could mean a radically different thing tomorrow. For this reason, data has to be processed in real-time because the results can be outdated the next morning or even the next second.
- Visualization: a way to present. Once data is processed, it must be demonstrated in a comprehensible manner. To visualize data, you need complicated graphs with a number of variables that still have to be readable for the audience.
How to use big data analytics in marketing
Big data is used in various industries like banking, communications, healthcare, and others. It's increasingly used in digital advertising and data-driven marketing to understand the target audience better. According to Forbes, 48% of marketers use big data for customer analytics, and it presents significant business opportunities.
Benefits of using big data analytics for marketers:
- Collect data about customers, understanding who they are, what they like, and what they need, which ultimately leads to a 360-degree view of the consumers;
- Create highly detailed profiles of the target audiences with the necessary attributes, such as demographics, geolocation, interests, hobbies, devices, social status, and others;
- Understand the patterns of online and offline consumer behavior, which allows for optimizing advertising campaigns in real time;
- Identify the causes of marketing failures and find optimal solutions with predicted outcomes;
- Improve customer engagement and leverage the ability to deliver personalized, highly targeted advertising messages at the right time, place, and context;
- Monitor the performance of the marketing campaign in real time and track consumer engagement levels;
- Plan and launch high-performance advertising campaigns that bring considerable revenues.
Challenges of big data analytics
Data volume and complexity
Dealing with large volumes of complex data in big data analytics can be overwhelming and expensive due to the need for advanced infrastructure and technologies.
Data quality
Data quality is essential for accurate analysis, but big data often contains irrelevant or incomplete information. Poor-quality data can result in misleading conclusions and bad decisions. Ensuring accuracy, consistency, and completeness requires ongoing monitoring and validation.
Data integration
Data in analytics on big data comes from various sources such as internal databases, social media, sensors, and third-party applications. Integrating this diverse data for analysis can be complex due to data silos and requires sophisticated tools and methodologies.
Conclusions
The importance of big data analytics is underscored by its ability to provide valuable insights, guiding businesses and brands to make informed decisions throughout every stage of their advertising campaigns. In order to transform raw data into actionable intelligence, it is crucial to utilize advanced big data analytics platforms and software. Leading providers such as IBM, HP, Microsoft, Dell, Teradata, and Oracle deliver comprehensive analytics solutions tailored to businesses of all sizes.
Unlock the full potential of your big data and discover the insights that can drive your success!
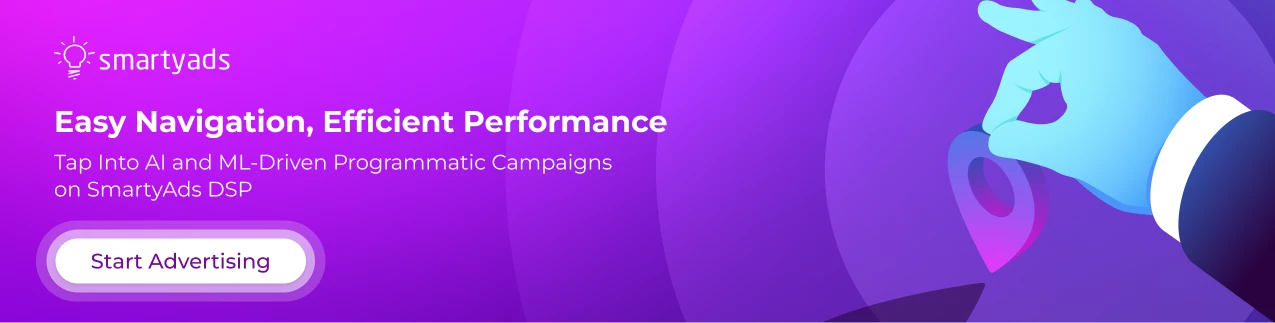